Independent Component Analysis based Color SIFT Descriptors for Image Classification
R, G, B channels in the RGB color space roughly follows the color receptors in the human eye, and are used in computer displays. Inspirited by the conception of color SIFT descriptors that calculates the descriptors for images in different color spaces, our second research focuses on finding an adaptive color representation for efficient feature extraction. On the one side, the existing color transformations transform the RGB color space into other color spaces based on physical models that are defined uniquely and are not dependent on input images. However, in some practical situations, training set has been already given in advance. Therefore, it is much better to take into account the object specific knowledge included in the training set, and impose it to SIFT descriptors. On the other side, R, G, and B channels are strongly correlated. It is necessary to transform input signals to reduce redundancy between inputs to follow the goal of vision information processing. Based on the above two aspects, our research proposes a novel color independent components based SIFT descriptors (CIC-SIFT). It is a two-step feature extraction method (see Fig. 1). In the first learning step, we learn a transformation matrix for each category using ICA. ICA, as a statistical method, is used to seek an adaptive color representation in which channels are as independent as possible. The second step is the color transformation step. We transform original images components into three independent components using the ICA-based transformation matrix. And then, the transformed color independent components are utilized to extract color SIFT features for object/scene classification (CIC-SIFT descriptor). Compared to conventional physical model-based color transformations, our proposed ICA-based transformation can be considered as an object-specific method which needs training set. It can enhance contrast between object and background in an image (see Fig. 2). Consequently, the proposed CIC-SIFT can extract more effective and discriminative local features for both object and scene classification.
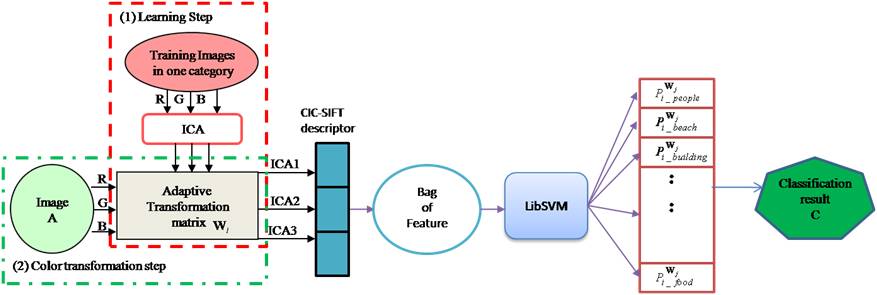
Fig. 1 Flowchart of object/scene classification by using CIC-SIFT descriptors
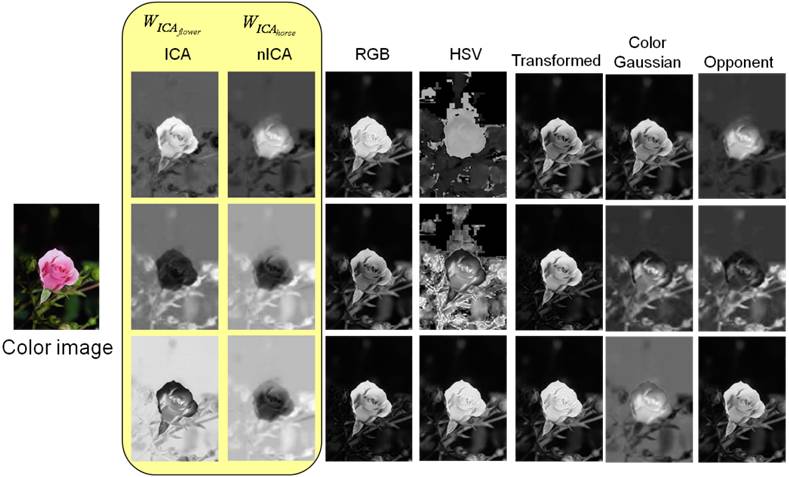
Fig. 2 Color space transformation
関連研究発表:
1. Dan-ni Ai, Xian-hua Han, Xiang Ruan and Yen-Wei Chen. Color Independent Components based SIFT Descriptors for Object/Scene Classification. IEICE Transactions on Information and Systems. Vol. E93-D, No. 9, pp. 2577 - 2586, Sep. 2010.
2. Dan-ni Ai, Xian-hua Han, Xiang Ruan and Yen-Wei Chen. Adaptive Color Independent Components based SIFT Descriptors for Image classification. The conference of the International Association for Pattern Recognition (ICPR2010). ThBCT9.11. Istanbul, Turkey. 2010.
3. Dan-ni Ai, Xian-hua Han, Xiang Ruan and Yen-Wei Chen. A Novel Color Independent Components based SIFT Descriptor. The Asia Simulation Conference 2009 (JSST 2009). P14. 2009.
4. Dan-ni Ai, Xian-hua Han, Guifang Duan, Xiang Ruan and Yen-Wei Chen. Independent Components Selection of Color SIFT Descriptors for Image Classification. Technical Committee on Pattern Recognition and Media Understanding (PRMU), 2011.
5. Dan-ni Ai, Xian-hua Han, Guifang Duan, Xiang Ruan and Yen-Wei Chen. ICA vs PCA Color SIFT Descriptors for Scene Classification. Meeting on Image Recognition & Understanding, IS2-75, 2010.