Active Appearance Modelを用いた特徴点自動抽出
コミュニケーションにおいて顔情報を大いに活用している.
顔情報はその人物の顔の形状,目や口などの器官形状,肌の色などに基づいて,性別,年齢,人種等などの違いを表現し,
人物特定や認証を行う.そのためにも顔情報解析の精度向上はより重要な課題である.
本研究では,顔の形状とテキスチャの両方を表現できるActive Appearance Model(AAM)を視点ごとに作成し,
各視点において正確に顔の特徴点を自動的に抽出することができる.
AAMの流れを図1に示す.
顔の形状バリエーションを表す統計形状モデルを図2に,テキスチャのバリエーションを表すテキスチャモデルを図3に示す。
また,AAMを用いて抽出した顔特徴点の例を図4に示す。
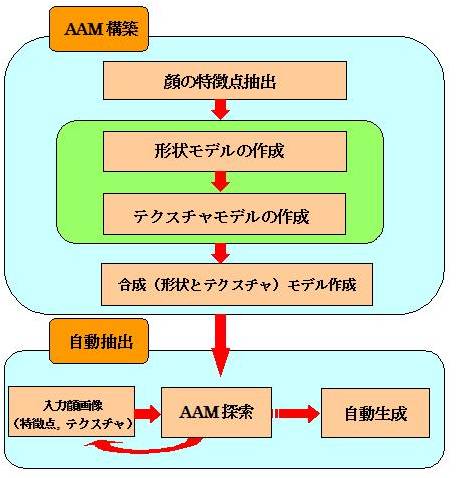
図1 Active Appearance Model (AAM)の処理の流れ
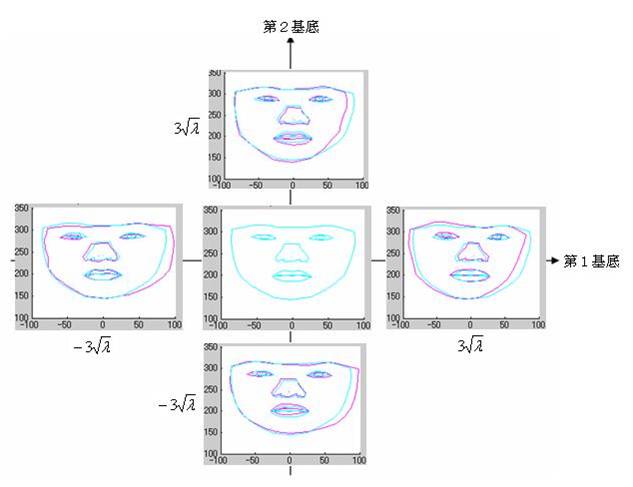
図2 形状モデル(第1基底と第2基底の変化量)
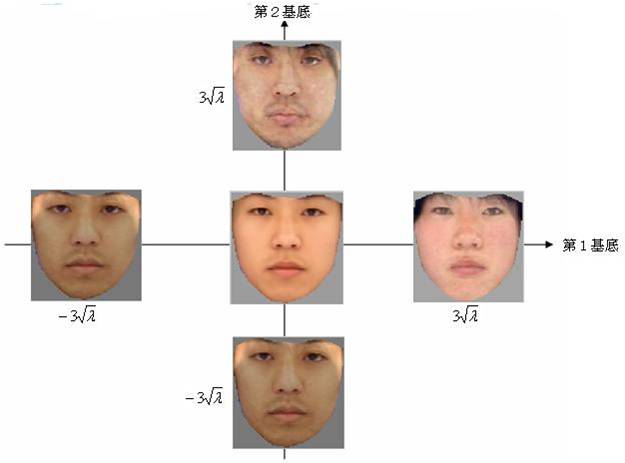
図3 テクスチャモデル(第1基底と第2基底の変化量)
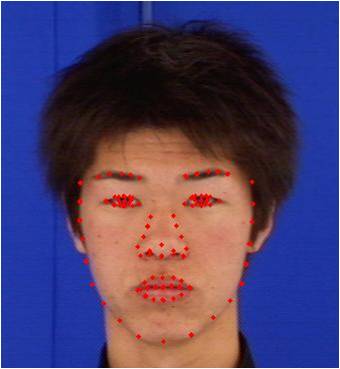
図4 特徴点自動抽出結果