Automatic Generation of Eye Gaze Corrected Video Using Recursive Conditional Generative Adversarial Networks
Eye contact plays an important role in conversations. However, it is difficult to maintain eye contact while using current popular video calling systems due to the different positions of the camera and display. To solve this problem, we introduce convolutional long short-term memory, which captures the features of frames up to the previous instant, into a deep learning generation model—conditional generative adversarial networks (GANs), which is recursive GANs—to generate eye gaze corrected video. By extending this network, the generator generates an image that takes the previous frame into account and the discriminator identifies the image by considering the previous frame. Thus, we aimed to achieve a consistent video conversion.
This work is a joint research with Samsung Japan Research Institute.
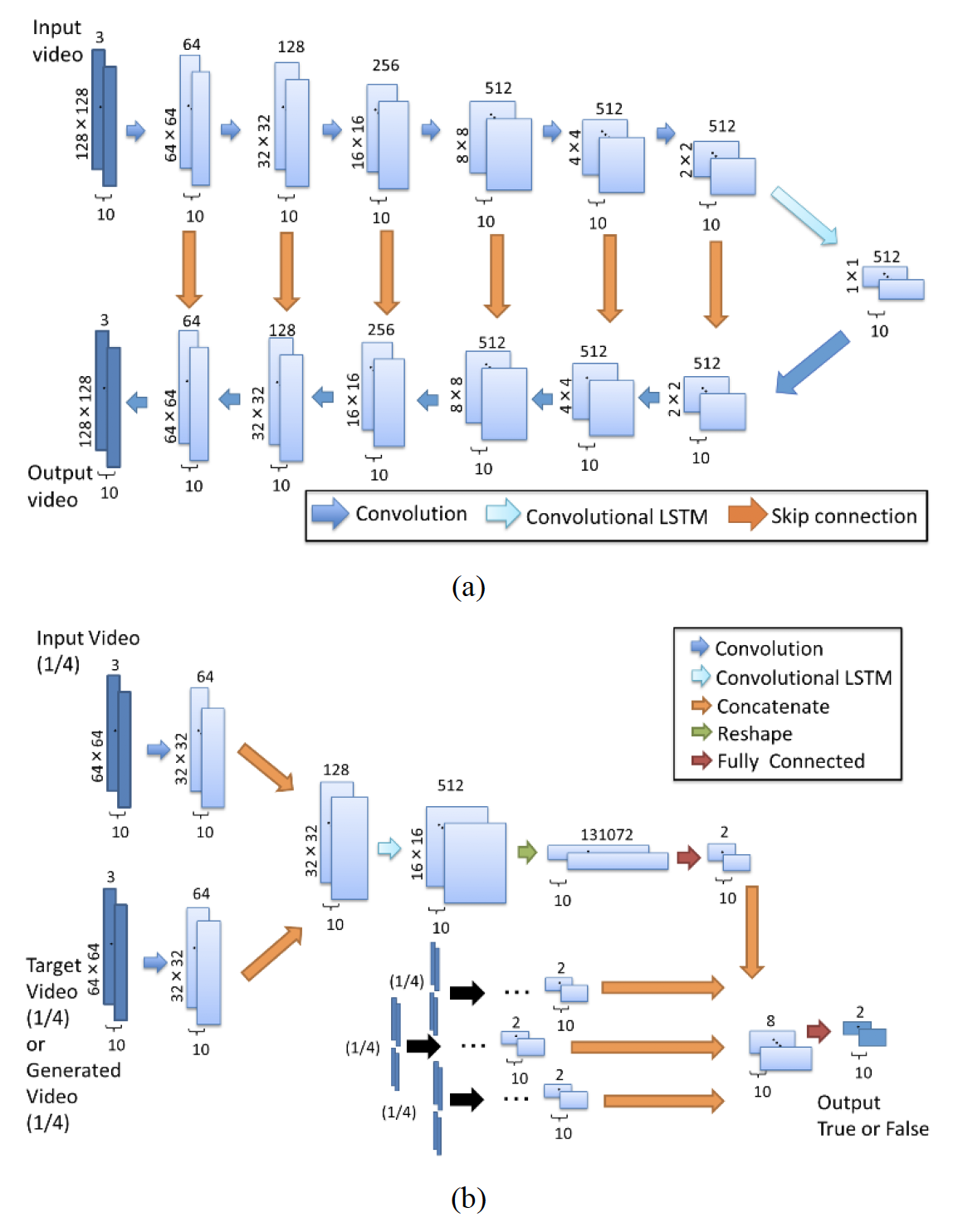
Fig.1. The proposed recursive conditional generative adversarial network(a) Ganerator; (b) discriminator.
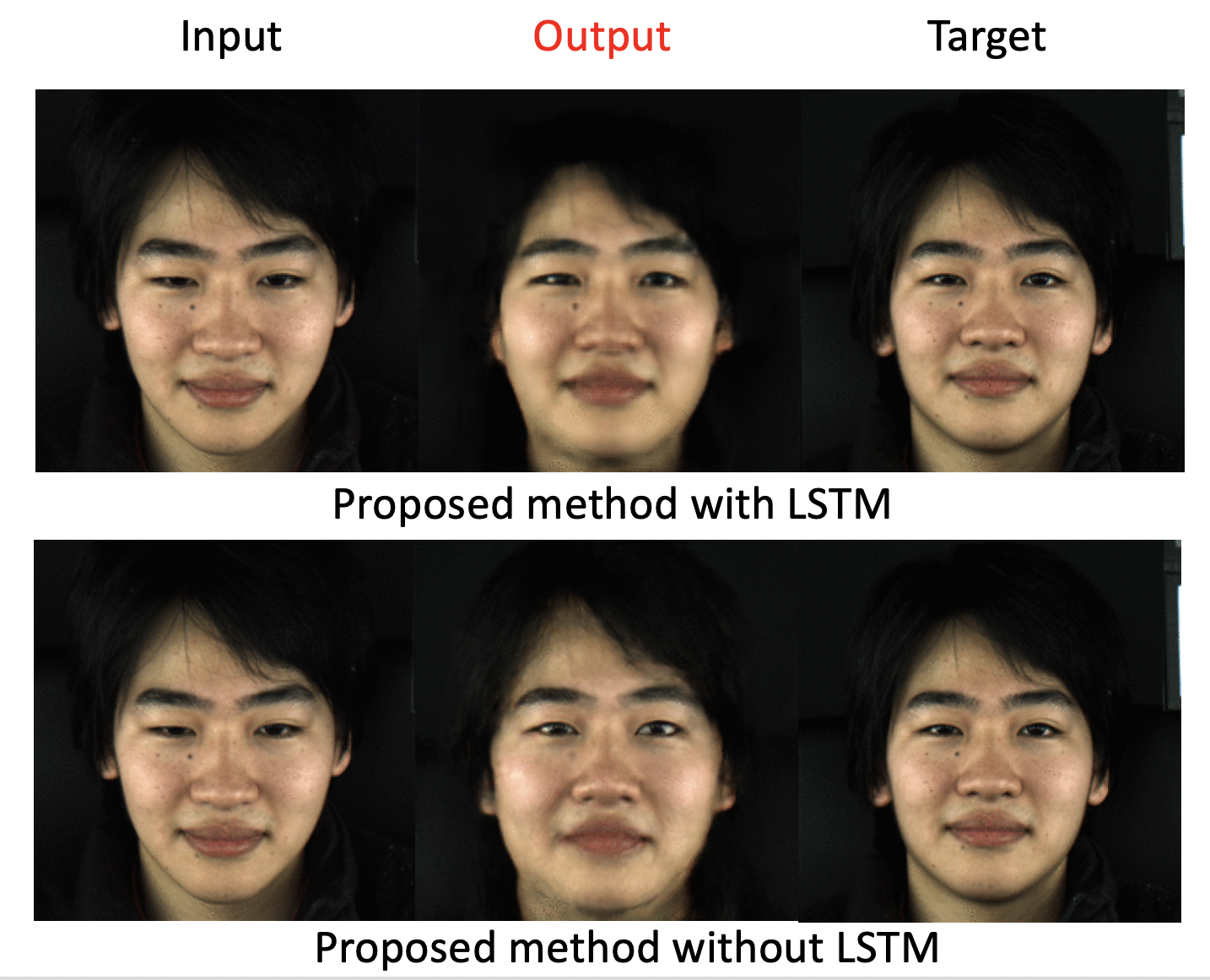
Fig.2. Eye gaze correction results
Related Publications:
1. Kento Otsu, Masataka Seo, Toshihiro Kitajima, Yen-Wei Chen, “Automatic Generation of Eye Gaze Corrected Video Using Recursive Generative Adversarial Networks,” Proc. of 2020 IEEE 8th Global Conference on Consumer Electronics (GCCE 2020), Kobe, Japan, Oct.12-14, 2020(Link)
2. Takahiko Yamamoto, Masataka Seo, Toshihiko Kitajima and Yen-Wei Chen, “Eye Gaze Correction Using Generative Adversarial Networks,” Proc. of 2018 IEEE 7th Global Conference on Consumer Electronics (GCCE 2018), Nara, Japan, Oct.9-12, 2018.(Link)