Facial Pose Synthesis with One 2D Image
When we obtain a person's profile facial image, can we generate this person's frontal facial image or other poses?
The method to solve this kind of problem is properly called "facial pose synthesis".
The essential idea of image synthesizing is extracting information from existing images and generating an accurate and detailed facial model.
It has been an active topic in computer vision, computer graphics and related fields.
Facial pose synthesis has a number of useful applications, such as for social security and cosmetology.
In social security, the facial image synthesis method can be applied to assist law enforcement.
Sometimes, due to the limits of circumstances, the police take suspect photos with some feature parts,
such as half of the face, invisible.
It is difficult for the police to recognize the suspect without having front facial information.
Facial pose synthesis techniques will help the police generate a frontal facial portrait and other poses.
It can also be applied into the field of cosmetology for skin appearance.
If one human nature facial image is obtained,
the person's cosmetic facial images under some conditions (such as mutative illuminations,
mutative view-angles) will be generated by using synthesis methods.
We propose a tensor-based subspace learning method (TSL) for facial pose synthesis.
We organize two-dimensional multi-pose images as a tensor form and apply tensor decomposition to build a projection subspace. In the synthesis process,
the input two-dimensional image is projected into the corresponding projection subspace to get an identity vector.
The identity vector is then used to generate other novel pose images.
This is motivated by the fact that Vasilescu and Terzopoulos have noticed that the tensor decomposition method is an efficient tool for feature detection,
which plays an important role in the synthesis learning method.
The method based on tensor decomposition has also been applied to synthesize facial expressions,
in which the authors only need a frontal facial pose and does not consider the differences in the same person's facial contour in different poses.
Compared with the morphable three-dimensional face model, our proposed method constructs a statistical model by training two-dimensional multi-pose images instead of three-dimensional scans and doesn't need any model fitting processing.
So it is easier to implement and can be used for on-line processing.
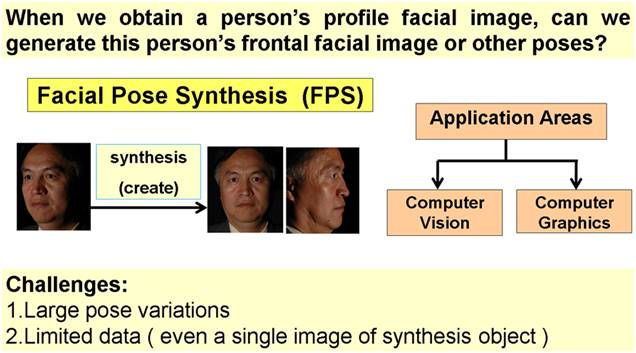
Figure 1. Purpose for Facial Pose Synthesis
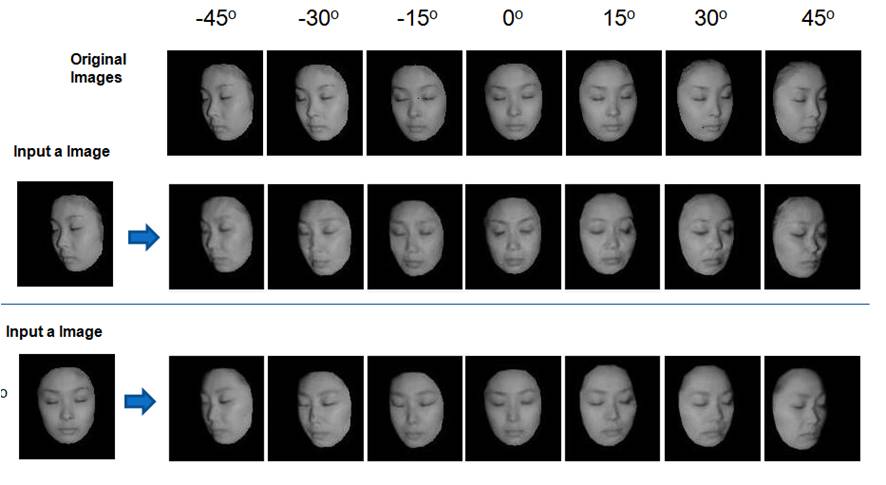
Figure 2. Synthesized Results
関連研究発表:
1. (Journal) X. Qiao, X. H. Han, T. Igarashi, K. Nakao, and Y. W. Chen. Tensor-Based Subspace Learning and Its Applications in Multi-Pose Face Synthesis, Neurocomputing, vol. 73, pp.2727-2736, 2010.
2. (Conference) X. Qiao, X. H. Han, Y. W. Chen, T. Igarashi, and K. Nakao. Synthesis of Multiple Pose Facial Images Using Tensor-Based Subspace Learning Method, 12th IEEE International Conference on Computer Vision (ICCV'09) on Subspace Workshop, pp.219-226, Kyoto, 2009.